Member-only story
NumPy Illustrated: The Visual Guide to NumPy
Brush up your NumPy or learn it from scratch
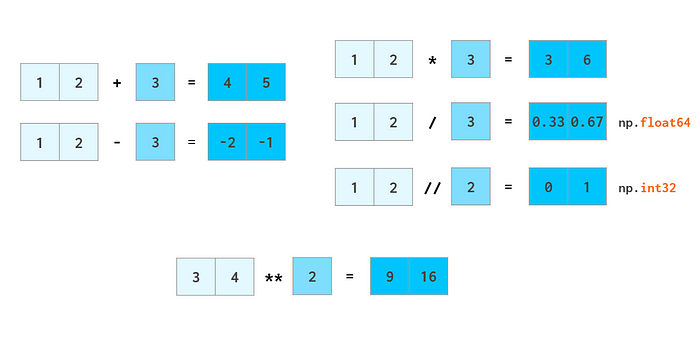
NumPy is a fundamental library that most of the widely used Python data processing libraries are built upon (pandas, OpenCV), inspired by (PyTorch), or can efficiently share data with (TensorFlow, Keras, etc). Understanding how NumPy works gives a boost to your skills in those libraries as well. It is also possible to run NumPy code with no or minimal changes on GPU¹.
The central concept of NumPy is an n-dimensional array. The beauty of it is that most operations look just the same, no matter how many dimensions an array has. But 1D and 2D cases are a bit special. The article consists of three parts:
I took a great article, “A Visual Intro to NumPy” by Jay Alammar², as a starting point, significantly expanded its coverage, and amended a pair of nuances.
Numpy Array vs. Python List
At first glance, NumPy arrays are similar to Python lists. They both serve as containers with fast item getting and setting and somewhat slower inserts and removals of elements.
The hands-down simplest example when NumPy arrays beat lists is arithmetic:

Other than that, NumPy arrays are:
- more compact, especially when there’s more than one dimension
- faster than lists when the operation can be vectorized
- slower than lists when you append elements to the end
- usually homogeneous: can only work fast with elements of one type

Here O(N) means that the time necessary to complete the operation is proportional to the size of the array (see Big-O Cheat Sheet³ site), and O*(1) (the so-called “amortized” O(1)) means that the time does not generally…